Research & Statistical Consulting
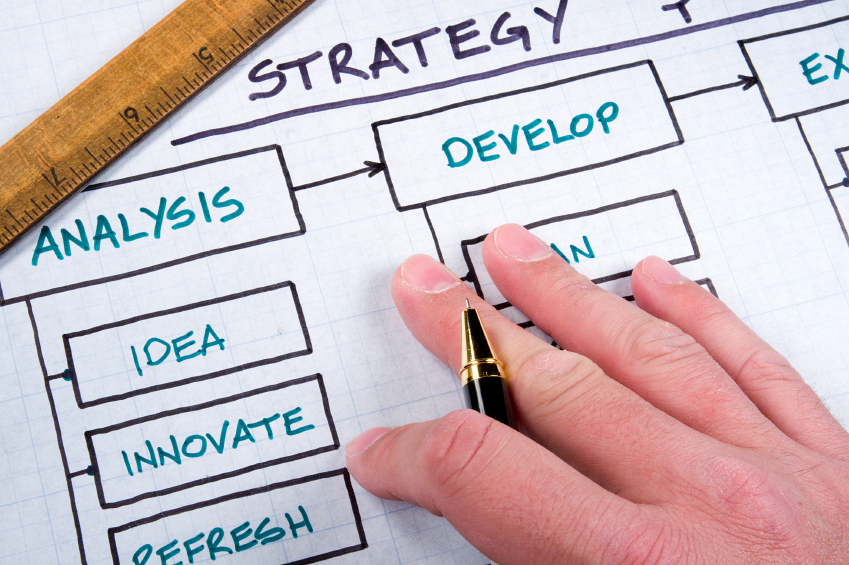
- Descriptive statistics
Mean, Mode, Median, Min, Max etc. by categories
- Graphical presentation of survey data
bar chart, lines, xy scatter plot and other univariate and multivariate presentation styles
- ANOVA, MANOVA, ANCOVA, MANCOVA, and GLM analyses
analysis of variance and covariance for single variable and multiple variables, one-way, two-way and more, with interactions, variance component and more …
- Multiple imputation techniques to replace missing values and missing data, to improve modeling reliability
This technique allows the use of observations with missing data based on the distribution of the data and random selection techniques
- Regression analysis, linear regression, linear probability models: logit, probit, binomial, multinomial
All sorts of regression models with OLS and non-linear such as probability models with ordinal or categorical outcomes, also response variable with Poisson or NBD distribution.
- Analysis of multi-level (Random Coefficient Models) data
data organized in two or more levels, Hierarchical data analysis with variables that vary at level one or vary at higher levels using HLM, MPlus or SPSS
- Longitudinal data (Individual Growth Curve Models), measuring random and fixed effects (unstructured, structured, etc.)
The analysis of multi-level data is important for data which has various sources of variance. The multi-level analysis treats levels of data separately and provides the variance explained by each level. This is extremely useful when data were repeatedly measured over time.
- Dyadic analysis of variations within and between associated couples
A new approach to analyzing data that come from coupled sources such as a married couple, twins, brothers and sisters, etc.
- Exploratory and confirmatory factor analysis, factor measurements (independently or within structural equation models)
Data reduction techniques to reduce number of similar variables by building unobserved factors
- Cluster analysis, unsupervised grouping
The cluster analysis combines observations into groups based on the similarities and differences between them
- Structural equation models that test sets of complex hypotheses where both direct and indirect effects are measured
The structural equation model runs several regression equations simultaneously to test complex hypotheses with mediation, moderation and interaction effects
- Mediation and moderation analyses of observed and unobserved instruments
regression models that assume an indirect effect of instrumental variables on the relationship between the dependent variable and the explanatory set of variables
- Power analysis, confidence interval and effect size
examining the validity of the data and the validity of the tests and the predictiability of the results